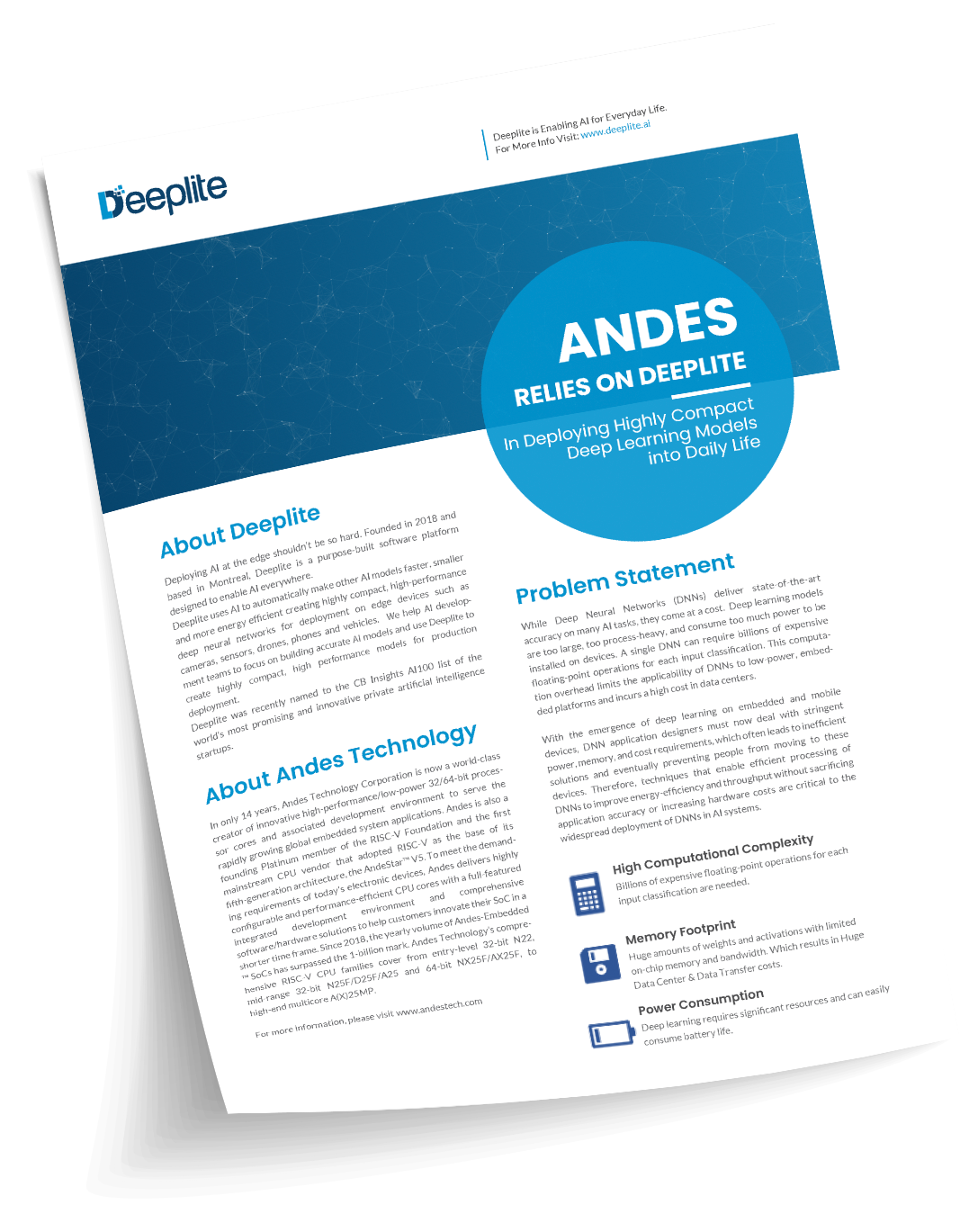
Andes Relies on Deeplite
In Deploying Highly Compact Deep Learning Models
Deep Neural Networks deliver state-of-the-art accuracy on many AI tasks, but they come at a cost. They are large, process-heavy, and consume too much power to be installed on devices. This computation overhead limits the applicability of DNNs to low-power, embedded platforms.
Learn how Andes and Deeplite worked together on a person detection use case, using low-power RISC-V MCU DSP (<256KB SRAM). Deeplite result was 2.7% more accurate and 15% faster than TensorFlow Lite Micro!
Fill in the form below to download the full case study.